Identifying Low-Effort Survey Responses: A Guide and App
Survey data impacts some of the most important decisions in higher education, with institutions relying on it to accurately capture the voices of their community. While many IR/IE offices are well aware of challenges in achieving high survey quality, one vital aspect often goes unnoticed: identifying (and removing) low-effort responses that compromise data integrity.
A lesser-known fact is that most leading survey organizations do not address these responses. Instead, they deliver raw, unfiltered data directly to IR/IE offices, leaving the responsibility of ensuring data quality squarely on the professionals tasked with reporting and analysis.
What Is Low-Effort Survey Responding?
Low-effort survey responses can take different forms. A low-effort survey taker might:
- Rush through the survey at an impossibly fast pace.
- Choose the same response option repeatedly.
- Stop answering questions.
- Provide unrealistic answers.
- Give bogus responses (i.e., wrong information).
There’s no universal formula or absolute standard for what makes a response “low-effort.” However, as IR/IE professionals, we can set reasonable, clear benchmarks to ensure the data we use is as accurate as possible.
3 Metrics You Can Use to Detect Low-Effort Responses
Here are three straightforward metrics and methods to identify low-effort responses:
- Survey duration: Completion time is one of the most reliable indicators of response quality. If someone finishes a survey in a fraction of the time it takes to answer thoughtfully, it’s a clear red flag. To set a reasonable benchmark, try taking the survey as quickly as you can while still answering meaningfully. Popular survey tools such as Qualtrics and SurveyMonkey have built-in tools to track survey duration.
- Straight-lining: Straight-lining occurs when respondents select the same answer across a series of questions (e.g., on Likert items). This method is somewhat difficult to implement in Excel (or R, via careless package), but can often identify a sizeable chunk of low-effort responses.
- Incorrect or unrealistic responses: Outlandish answers—like claiming 25 hours of daily activity—are clear indicators of low effort. These questions are often survey-specific, and you can set plausible limits to help flag these responses. Another example is when a student’s actual characteristics, such as major, class level, or race/ethnicity, do not match their survey responses.
While these three methods will not “catch” every low-effort response, they will undoubtedly help improve your survey quality. For other techniques for identifying low-effort responses, such as detecting complex response patterns, visit my additional resources.
Introducing An App
To make the process of identifying low-effort responses more accessible, I’ve developed a secure, user-friendly app tailored to IR professionals. Currently designed for NSSE data (with plans to expand to other surveys), the app:
- Flags low-effort responses based on duration, skipped questions, straight-lining, and implausible answers.
- Provides detailed explanations for flagged entries, empowering you to make informed decisions.
- Exports annotated datasets so you can integrate this analysis into your workflow.
Key tabs include the following:
- Summary Tab: Displays flagged responses categorized by issues like completion speed, missing answers, straight-lining, and implausible data.
- Explore Data Tab: Shows examples of flagged responses and why they were flagged.
- Download Data Tab: Allows you to export your dataset with added columns that indicate why you should—or shouldn’t—remove these respondents from analysis.
To use the app, upload a raw data file of your students’ responses that is provided by NSSE.
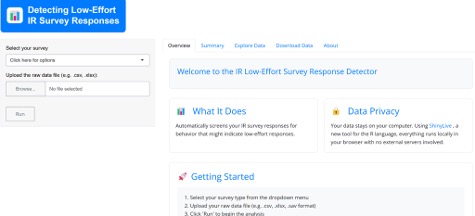
Dr. Steven Sherrin is Senior Researcher II at Wentworth Institute of Technology. If you’d like to suggest a survey for the app, refine the methodology, or share your own insights, you can contact him at stevensherrin@gmail.com.